Quantitative Assessment in Forestry: Methods and Applications
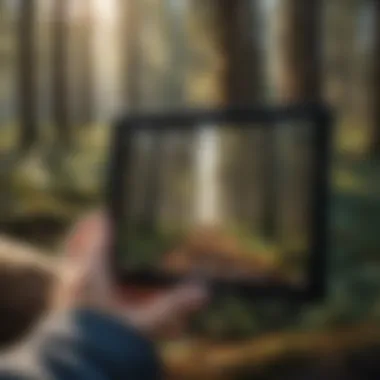
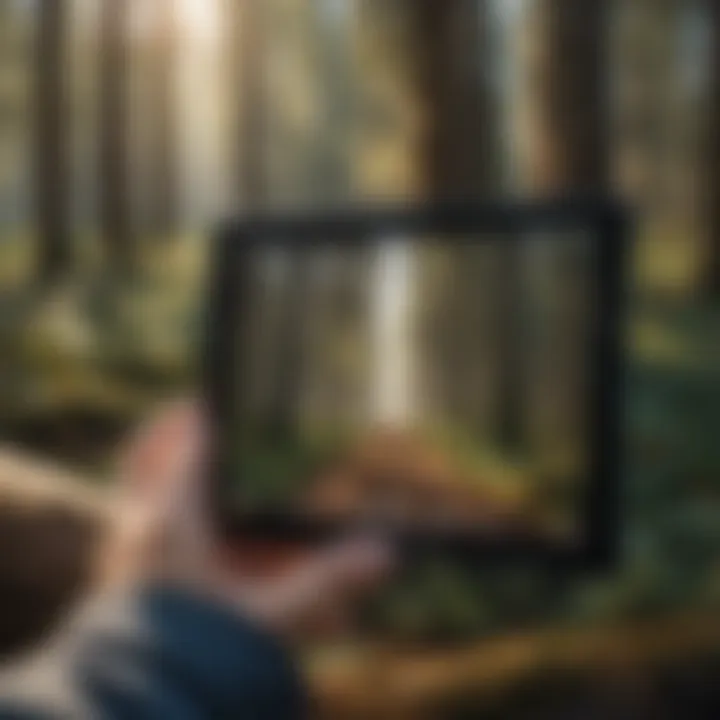
Intro
Quantitative assessment in forestry has become paramount in understanding and managing forest ecosystems effectively. This focus on numbered metrics allows professionals to gauge forest health, estimate biomass, and optimize resource management strategies. With the rise of statistical models and data analysis tools, forestry is entering a new era of precision and sustainability.
Overview of Forestry Practices
Forestry practices encompass a wide range of activities that are crucial for maintaining ecological balance and supporting economic needs. Understanding these practices is essential for anyone involved in forest management.
Importance of Foresting
Forests play a vital role in our environment. They provide habitat for wildlife, contribute to carbon sequestration, and offer recreational spaces for communities. Proper management ensures these benefits are sustained for future generations. Key reasons include:
- Biodiversity Preservation: Forests are home to numerous species, many of which are endangered.
- Climate Regulation: They regulate temperature and maintain the water cycle.
- Economic Resources: Sustainable foresting practices provide timber and non-timber resources.
Types of Forestry Practices
Forestry can be categorized based on goals and techniques used:
- Sustainable Forestry: Balances ecological health and economic viability.
- Agroforestry: Integrating agriculture with forestry to maximize land use.
- Community Forestry: Ensures local communities manage and benefit from forests.
Best Practices for Sustainable Woodland Stewardship
Sustainable woodland stewardship is essential in safeguarding our forests. Practices that promote long-term health and productivity need to be prioritized.
Principles of Sustainable Forestry
- Ecosystem Services: Recognizing the range of benefits forests provide is crucial.
- Adaptive Management: Flexibly adjusting practices based on ongoing assessments ensures resilience.
- Inclusivity: Engaging local communities in decision-making enhances sustainability efforts.
Techniques for Land Management
Effective land management practices involve a mix of traditional knowledge and modern science. Some techniques include:
- Selective Logging: Minimizing impact on the forest structure while harvesting.
- Reforestation: Planting trees in deforested areas to restore ecosystems.
- Controlled Burns: Used to reduce hazardous fuel load while benefiting some tree species.
Innovative Techniques in Forestry
With rapid technological advancements, innovation in forestry is not just beneficialβit is necessary. Emerging techniques can lead to improved results in assessments and sustainable practices.
Latest Technological Advancements
- Remote Sensing: Satellite imagery and drones allow for precise monitoring of forest health.
- GIS Technology: Geographic Information Systems enhance spatial data analysis for better decision making.
- Data Analytics: Big data tools play a critical role in understanding trends and patterns in forestry.
Integrating Traditional Knowledge with Modern Science
Combining local wisdom with technological insights can lead to more effective forest management. Traditional practices often provide tried and tested methods that complement scientific approaches. For instance, indigenous fire management techniques can coexist with modern forest fire prevention measures.
"Integrative approaches in forestry amalgamate traditional practices with modern techniques, enriching the overall assessment and management outcomes."
Continuing to refine and apply quantitative assessment methods is key to the future of forestry. Understanding the available techniques and the significance behind them can empower professionals and enhance the sustainability of forest ecosystems.
Prolusion to Quantitative Assessment
Quantitative assessment in forestry is essential for evaluating various dynamics within forest ecosystems. The methods used to measure and analyze data provide insightful information that aids in sustainable forest management. Understanding quantitative assessment allows forestry professionals to implement strategies based on data rather than assumptions, which is vital for ecosystem conservation and resource management.
Definition and Importance
Quantitative assessment refers to systematic measurements that yield numeric data regarding various aspects of forestry, such as tree growth, biomass estimation, and the overall health of a forest. This data-driven approach offers a reliable basis for decision-making and policy formulation. The importance of these quantitative methods cannot be overstated; they offer insights into population dynamics, resource availability, and potential impacts of environmental changes. Moreover, effective application of quantitative methods leads to more accurate predictions and efficient resource allocation, which ultimately supports environmental sustainability.
Historical Context in Forestry Studies
The concept of quantitative assessment is not new in forestry; it has evolved over decades of research and application. In the early days, forestry studies relied predominantly on qualitative assessments. However, as the field progressed, the need for precise measurements became evident. The introduction of statistical methods in the 20th century marked a significant turning point. Researchers began employing techniques such as regression analysis and sampling theory to enhance the reliability of their findings. This shift to quantitative studies fostered a more rigorous understanding of forest dynamics, ecology, and conservation strategies. Today, advancements in technology and data analysis have further refined these methods, paving the way for comprehensive evaluations and more informed management practices.
"Quantitative methods have revolutionized forestry studies by introducing data-driven insights that were previously unattainable."
Understanding the historical context of quantitative assessments helps in grasping their current applications and the ongoing developments within the field. It illustrates how effective methodologies have shaped contemporary forestry practices and highlights the necessity for continuous improvement and adaptation to new challenges.
Key Concepts of Quantitative Assessment
Quantitative assessment in forestry plays a vital role in understanding and managing forest ecosystems. By employing various quantitative methods, forestry professionals can gain insights into forest health, biomass, and resource allocation. The precise evaluation of these factors supports sustainable forest management, ensuring that both ecological integrity and economic viability are maintained.
Statistical Methods
Descriptive Statistics
Descriptive statistics focuses on summarizing and organizing data. This method enables researchers to present the basic features of data effectively. One significant aspect is its ability to provide simple summaries about sample and measures. This includes averages, median values, and variability, which are critical for understanding forest metrics.
Descriptive statistics is popular because it offers clear, directly interpretable results, which are essential in forestry research. A key characteristic of this method is its reliance on visualizations such as graphs and charts, making complex data more digestible. However, its limitation lies in not drawing conclusions or making predictions beyond the immediate data. This method solely describes data without implying trends or causes.
Inferential Statistics
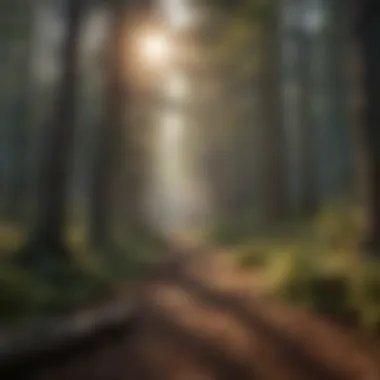
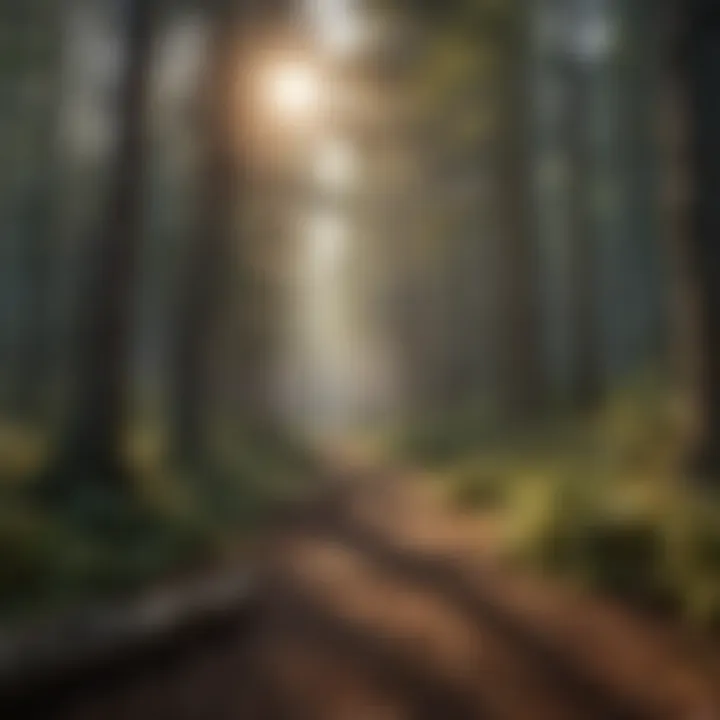
Inferential statistics extends the concepts of descriptive statistics by allowing researchers to make predictions or inferences about a population based on sample data. This method is crucial in forestry, as it can help estimate tree population sizes or predict the impact of certain variables on forest health.
The uniqueness of inferential statistics lies in its use of probability distributions, confidence intervals, and hypothesis testing. By leveraging these characteristics, statistics helps ensure data extrapolations are statistically valid, which is a valuable tool in ecological studies. Nonetheless, the assumptions required can sometimes lead to misinterpretations if the sample data is biased or not representative of the target population.
Data Collection Techniques
Remote Sensing
Remote sensing involves collecting data from a distance, typically through aerial or satellite imagery. This technique significantly enhances the quality of quantitative assessments by providing comprehensive views of forest health over vast areas without the need for intrusive ground surveys. It contributes to understanding changes in land use, vegetation cover, and deforestation rates, making remote sensing a critical tool for contemporary forestry management.
A notable characteristic of remote sensing is its capability to capture multispectral data, allowing detailed analysis of vegetation health and biomass. This feature makes it a popular choice among forestry professionals. However, potential downsides include high costs of advanced satellite imagery and the requirement for specialized training to interpret complex data.
Field Surveys
Field surveys involve direct collection of data from forest environments. This method provides in-depth, real-time information about local terrain, species diversity, and tree measurements. Its contribution to quantitative assessments is substantial, as it allows for the acquisition of quality data that reflects current conditions accurately.
The key strength of field surveys is their adaptability to various environments, making them useful for specific site assessments. Such surveys are often more cost-effective than remote sensing, particularly in smaller areas. On the other hand, field surveys can be time-consuming and labor-intensive, potentially affecting the quality and breadth of data if not conducted meticulously.
The integration of statistical methods and data collection techniques offers a robust framework for effective quantitative assessments in forestry, essential for sustainable management practices.
Biomass Estimation Techniques
Biomass estimation is a crucial part of forestry research. It helps in understanding forest structure, function, and health. Assessing biomass accurately allows forest managers to make informed decisions about resource management and sustainability. When focusing on biomass estimation methods, two approaches stand out: allometric equations and direct measurement methods. Both have distinctive roles and applications.
Allometric Equations
Allometric equations are relationships between the size of trees and their biomass. These equations typically use easily measurable tree parameters like diameter at breast height (DBH) or tree height. For instance, a common allometric equation is one that estimates biomass based on DBH alone, which simplifies data collection. Researchers favor allometric equations due to their efficiency, especially in large-scale forest assessments.
However, it is essential to consider that allometric equations can vary based on species and environmental conditions. That means applying the wrong equation may lead to inaccurate estimates. To address this issue, it is crucial to develop equation models based on local data and tree species. This adaptation enhances the reliability of biomass estimates and provides more relevant data for managers.
Direct Measurement Methods
Direct measurement methods offer a hands-on approach for estimating biomass. This technique involves measuring specific biomass components, like leaves, stems, and roots. While this method can provide precise measurements, it is often labor-intensive and time-consuming. It is primarily used in smaller or experimental plots.
One popular approach within direct measurement is the destructive sampling method. This involves harvesting a tree or a part of it to measure the biomass accurately. Though this provides accurate data, it limits the number of trees that can be assessed and may interfere with ecosystem dynamics.
Forest Health Assessment
Forest health assessment plays a crucial role in understanding the condition and sustainability of forest ecosystems. This assessment involves examining various indicators and parameters that reveal the state of a forest, which in turn can inform management practices and conservation efforts. By systematically evaluating forest health, professionals can identify areas of concern, monitor changes over time, and implement strategies to enhance forest resilience.
One of the specific benefits of conducting a forest health assessment is the ability to detect hazards that threaten forest ecosystems. These can include pest infestations, diseases, and the impacts of climate change. Early detection of these issues allows for timely intervention, maintaining the forest's biodiversity and ecological function. Additionally, healthy forests contribute to carbon sequestration, water purification, and soil protection, which are vital for combating climate change and supporting local communities that rely on forest resources.
Several considerations are essential when carrying out a forest health assessment. First, it is necessary to select appropriate indicators that accurately reflect forest conditions. Also, using standardized methods for data collection and analysis enhances the reliability of the results, making it easier to compare assessments over time or across different landscapes. Furthermore, incorporating local knowledge and stakeholder input is vital, as it can provide insights into specific challenges faced by the forest being assessed.
Indicators of Forest Health
Indicators of forest health are measurable variables that provide insight into the state of the forest. These indicators can cover various elements, such as tree growth, species diversity, and the presence of invasive species.
Some important indicators include:
- Tree Vitality: Assessing tree health can involve examining leaf color, crown density, and overall growth rates. Healthy trees typically exhibit lush foliage and robust growth patterns.
- Species Diversity: A diverse mix of species can indicate a resilient ecosystem. High species diversity often correlates with improved disease resistance and greater adaptability to environmental change.
- Soil Quality: Soil health is a key indicator of forest vitality. Parameters such as organic matter content, nutrient levels, and microbial activity give insights into soil ecosystem functions and energy cycling.
- Wildlife Presence: The variety and number of wildlife species can reflect the overall health of the forest, as many animals rely on specific habitat conditions tied to forest health.
By regularly monitoring these indicators, forest managers can make informed decisions aimed at improving or maintaining forest health.
Vitality Assessment Techniques
Vitality assessment techniques are methodologies employed to evaluate the health and robustness of forest trees. These techniques can vary in complexity and may include both field-based measurements and remote-sensing tools.
Key techniques include:
- Visual Inspection: Basic yet effective, visual inspections involve examining trees for symptoms of stress, such as discoloration, fungal infections, or damaged bark. This technique can quickly flag potential health issues for further investigation.
- Growth Measurements: Measurement of diameter at breast height (DBH), tree height, and crown expansion provides quantitative data on growth patterns, helping to identify trees that may be underperforming.
- Dendrometers and Tapes: These tools measure tree growth over time, capturing data on both diameter growth and height increment.
- Remote Sensing: Utilizing satellite imagery or drone technology can provide comprehensive data on forest cover, biomass estimates, and changes in health conditions across large areas.
Implementing these techniques in a systematic manner enables a well-rounded approach to assessing forest vitality.
Forest health assessment thus embodies a critical component of sustainable forestry practices, facilitating the ongoing monitoring and evaluation of forest ecosystems.
Application of Statistical Models
The application of statistical models in forestry plays a crucial role in understanding and managing forest ecosystems. These models provide quantitative insights into various aspects of forestry, such as growth patterns, yield predictions, and biodiversity assessments. Utilizing statistical approaches allows researchers and professionals to make informed decisions based on empirical data. The benefits of employing statistical models are significant.
First, these models enhance the ability to analyze complex data sets. They reveal underlying trends and relationships that may not be evident through basic observations. This deeper analysis supports better forest management practices and sustainability efforts. Additionally, statistical models can facilitate forecasting. For instance, they can predict future forest growth or potential impacts of market changes on timber supply.
Moreover, statistical models enable the integration of diverse data sources. By combining data from satellite imagery, field surveys, and historical records, forestry experts can develop more comprehensive assessments. These assessments help articulate the current health of forests and project future scenarios. Nevertheless, there are considerations to keep in mind. The choice of a statistical model should be based on the specific objectives of the study. Not all models are suitable for every situation, and careful evaluation is necessary to select the appropriate one.
In summary, the use of statistical models is instrumental in bridging gaps in knowledge and guiding policy decisions in forestry. The strength of these models lies in their ability to transform raw data into actionable insights, contributing to the sustainability and productivity of forest resources.
Regressional Analysis in Forest Studies
Regressional analysis is a powerful tool in the realm of forest studies. This method allows researchers to examine relationships between variables systematically. In forestry, it can be used to understand how different factors, such as soil quality, precipitation, and temperature, influence tree growth and forest health.
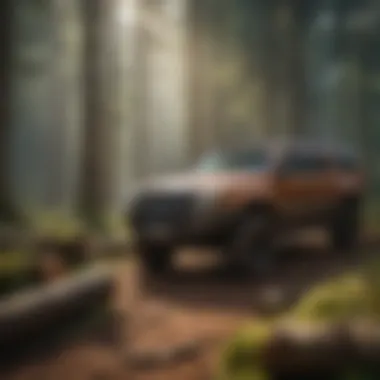
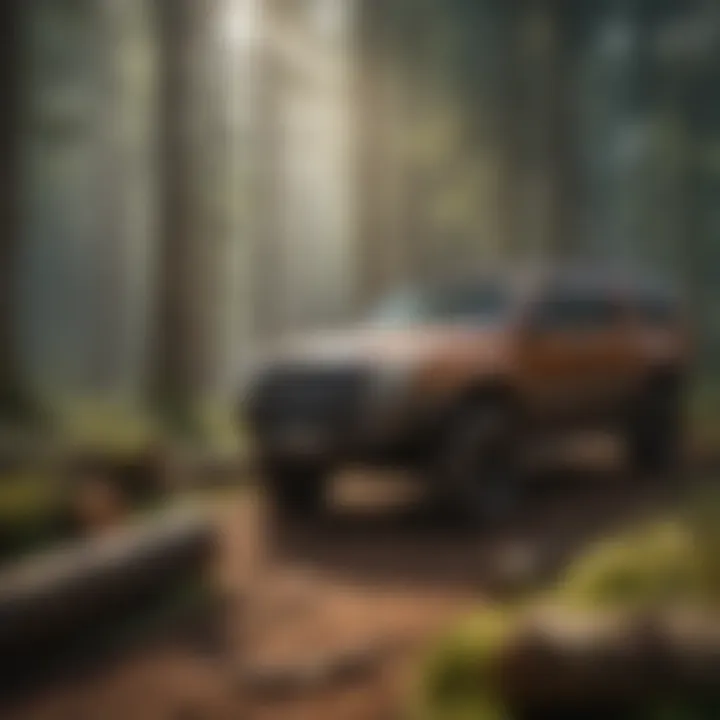
Through regressional analysis, it becomes feasible to quantify these relationships. This quantification provides a basis for predicting outcomes under various scenarios. For example, one may want to predict forest biomass based on a range of ecological variables. By applying regressional models, foresters can derive equations that describe these relationships. This information is invaluable for decision-making, particularly in resource management and conservation efforts.
Furthermore, regressional analysis supports hypothesis testing. Researchers can evaluate assumptions about ecological interactions and validate their findings with statistical evidence. This systematic approach further enhances the credibility of forestry research.
Machine Learning Techniques
Machine learning techniques have increasingly become a game changer in forestry assessments. These approaches utilize algorithms that learn from data, making predictions based on patterns identified in large datasets. One significant advantage of machine learning is its ability to handle vast amounts of data with relative ease. As forestry professionals strive to integrate diverse data sources, machine learning can automate analysis, streamlining the process of extracting insights from complex datasets.
For example, machine learning can classify different types of vegetation or detect changes in forest cover over time using satellite imagery. These insights aid in tracking deforestation rates or identifying areas that require conservation efforts. Moreover, machine learning simplifies the identification of potential risks to forest health by flagging anomalous patterns associated with pests or diseases.
However, successful application of machine learning techniques requires careful model selection and training. The choice of algorithm and the quality of the input data significantly affect the outcomes. Therefore, forestry professionals must remain diligent in refining their models and verifying the results.
Remote Sensing and GIS Applications
Remote sensing and Geographic Information Systems (GIS) are essential components in the domain of quantitative assessment in forestry. They provide powerful tools for data collection, analysis, and visualization of forest resources. By using these technologies, forestry professionals can gather spatial data that is critical to understanding forest dynamics and health.
Integration of GIS in Forestry
GIS allows for the integration of various data sources into a single framework. This enhances our ability to conduct comprehensive analyses and make informed decisions based on multiple variables. In forestry, GIS is utilized for mapping forest cover, analyzing habitat suitability, and assessing the impact of human activities on forest ecosystems. The ability to visualize data geographically adds a layer of understanding that traditional methods often lack. Furthermore, GIS facilitates the management of large datasets, enabling forestry professionals to track changes over time.
The benefits of integrating GIS in forestry include:
- Enhanced Decision Making: By presenting data visually, GIS supports better strategic planning and policy formulation.
- Resource Management: GIS assists in identifying areas that require conservation or restoration efforts, thus optimizing resource usage.
- Monitoring Changes: Regular updates to GIS data allow for real-time monitoring of forest conditions, aiding in prompt response to issues like disease outbreaks or invasive species.
Satellite Imagery for Quantitative Analysis
Satellite imagery is a key tool in effective quantitative assessment. It provides broad coverage and consistent data that is crucial for examining large forest areas. The periodic acquisition of satellite images helps track changes in forest cover, biomass, and health over time. Organizations like NASA and ESA offer satellite data that can be analyzed to provide insights into forest growth patterns, canopy structure, and even temperature variations in forested regions.
Some key applications of satellite imagery in forestry assessment are:
- Forest Cover Change Detection: Satellite data helps in identifying deforestation rates and reforestation efforts.
- Biomass Estimation: Remote sensing techniques can estimate forest biomass by measuring vegetation indices derived from satellite images.
- Health Assessment: By analyzing spectral data, satellite imagery can reveal areas affected by disease, pests, or environmental stressors.
Satellite imagery is a game changer, enabling unprecedented access to transversal data required for effective forest management.
The integration of these technologies in forestry not only improves accuracy but also enhances efficiency in data analysis and forest resource management. As the field evolves, continuing advancements in remote sensing and GIS applications are expected to further enrich quantitative assessment methodologies in forestry.
Challenges in Quantitative Assessment
In forestry, quantitative assessment tools offer valuable insights into forest health, biomass estimates, and resource management. However, these methodologies are not without their challenges. Understanding these obstacles is critical for forestry professionals and researchers. Addressing challenges is important to enhance the effectiveness of assessments and ensure sustainable forest management practices.
Data Quality and Availability
Quality data is the cornerstone of effective quantitative assessment. When data is scarce or of low quality, any analysis becomes unreliable. Issues such as missing data, inaccuracies, or outdated information can hinder the entire assessment process. In forestry, factors such as seasonal changes, varying geographic conditions, and ecological diversity can contribute to these data challenges.
The following elements are crucial to ensuring data quality:
- Consistency: Data should be collected using standardized methods. This practice assists in mitigating variability that can arise from different measurement techniques.
- Relevance: Selected data must align with the assessment goals. Irrelevant data can lead to misleading conclusions.
- Timeliness: Current data is essential for accurate assessments. Forest conditions change over time, so data must be up-to-date to reflect these changes effectively.
Access to data can also present hurdles. Many regions may lack comprehensive datasets due to budget constraints or insufficient research efforts. Collaboration among institutions could improve data availability. Through partnership initiatives, landowners, government agencies, and academic institutions can work together to gather and share vital information, thus enhancing the landscape of quantitative assessment in forestry.
Interpretation of Quantitative Data
Once data is collected, interpreting it correctly is paramount. Misinterpretation can lead to inaccurate conclusions, which may adversely impact forest management practices. Effective interpretation of quantitative data involves understanding both the statistical models used and the ecological context of the data.
Several considerations must be taken into account during the interpretation phase:
- Statistical Literacy: Understanding statistical methodologies is essential for forestry professionals. Familiarity with concepts such as confidence intervals and significance testing can influence decision-making.
- Ecological Context: Data does not exist in a vacuum. It is fundamental to consider environmental factors that might affect forest conditions. Ignoring these factors can lead to oversimplified conclusions.
- Complexity of Relationships: The relationships within ecological systems are often intricate. Care must be taken not to draw conclusions based on correlations that do not imply causation.
Effective interpretation of data enhances the reliability of quantitative assessments. The ability to communicate findings clearly to stakeholders is equally important.
In summary, the challenges associated with quantitative assessment in forestry primarily revolve around data quality and interpretation. Addressing these challenges requires an understanding of the fundamental aspects and a commitment to continuous improvement in practices. Through proactive strategies, forestry professionals can navigate these difficulties effectively.
The Role of Technology in Assessments
In recent years, technology has played an increasingly vital role in quantitative assessment within forestry. The integration of advanced tools has significantly transformed how data is gathered and analyzed. This is crucial for improving the accuracy of forest assessments and making well-informed management decisions. The application of technology goes beyond merely supporting existing methods; it enhances their efficiency and expands their capabilities, providing a more nuanced understanding of forest ecosystems.
Emerging Technologies in Forestry
Emerging technologies have the potential to revolutionize forestry practices. Drones, for example, are becoming widely used for aerial surveys. They offer an efficient and less intrusive means to gather data. Equipped with high-resolution cameras, drones can capture precise images of forested areas. This allows for the monitoring of vegetation health, species diversity, and biomass estimation without the need for extensive ground surveys.
In addition to drones, remote sensing techniques have shown notable advancements. LiDAR (Light Detection and Ranging) is one such technology that uses laser pulses to measure distances. This technique produces detailed three-dimensional models of forests that can reveal information about tree density, height, and volume. Through such data, forestry professionals can access insights that were once difficult to obtain, shaping strategies for carbon stock assessment and biodiversity conservation.
Moreover, Geographic Information Systems (GIS) have become instrumental in managing and analyzing forest data. GIS integrates various data types, allowing users to visualize and assess spatial relationships effectively. It supports sustainable forest management and land-use planning by helping identify areas at risk or those that require enhancement.
Impact of Technology on Data Analysis
The advent of technology impacts not only data collection but also its analysis. Sophisticated statistical software enhances the processing of large datasets. This allows for the implementation of complex statistical models that require heavy computational support. As a result, foresters can address multiple variables in their assessments, leading to more robust conclusions.
For instance, machine learning algorithms are increasingly being integrated into forest assessments. These algorithms can identify patterns in historical data, predict future scenarios, and assist in decision-making processes. When data analysis is automated, it allows professionals to focus on interpreting results rather than getting bogged down in data manipulation.
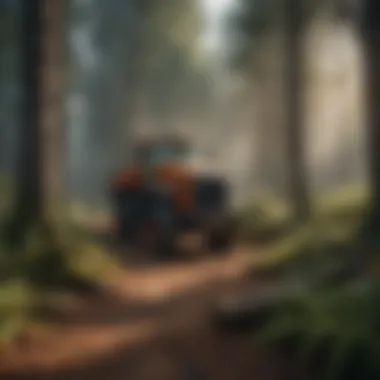
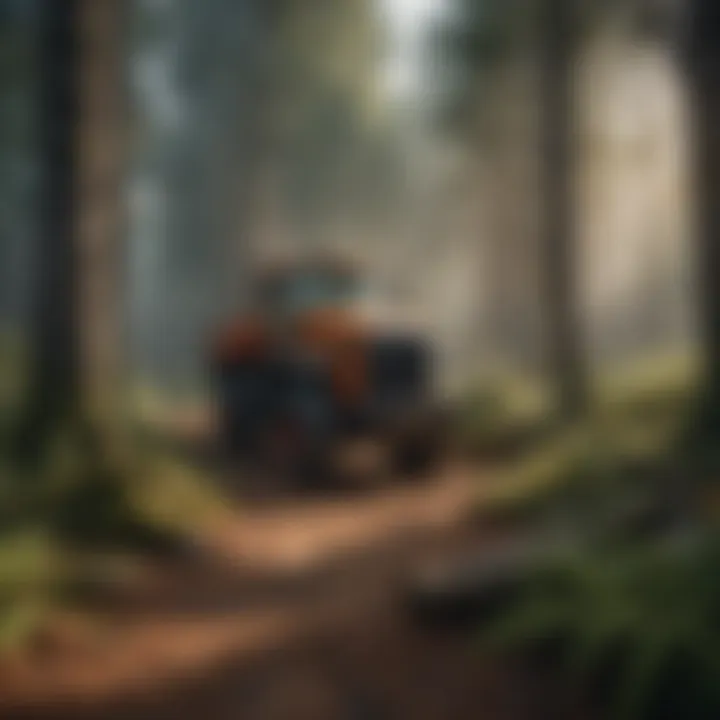
"The integration of technology in forestry is not merely beneficial; it is essential for informed decision-making."
Its significance cannot be overstated, as these advancements pave the way for better stewardship of forest resources.
Case Studies in Quantitative Assessment
Case studies serve as practical illustrations of theories and methodologies presented in quantitative assessment. They provide a real-world perspective, offering insights into the application and effectiveness of various techniques used in forestry. By examining specific instances of quantification methods employed in forest management, researchers and practitioners can understand the impact and significance of quantitative assessment in addressing complex forestry challenges.
Successful Applications in Forest Management
Successful applications of quantitative assessment can be seen in various forest management practices. One notable case is the application of allometric equations in biomass estimation. In regions such as the Amazon rainforest, researchers have utilized these equations to estimate the carbon content of trees end-to-end. This application not only provides an accurate measure of biomass for carbon trading but also aids in establishing forest conservation policies.
Another example involves the use of remote sensing technology combined with GIS data to monitor forest health. In the Pacific Northwest of the United States, land managers have employed such technologies to track changes in forest cover over time. The integration of remote sensing allows for large-scale assessments that are less invasive and more efficient than traditional field surveys.
Furthermore, cases highlight the role of statistical models in predicting forest growth and yield. Using regressive analyses, managers can simulate various management scenarios. This predictive power equips decision-makers with the information necessary to optimize resource allocation, leading to more sustainable practices.
Lessons Learned from Quantitative Studies
Analyzing case studies in quantitative assessment reveals several lessons that could benefit future research and application. First, the importance of data quality cannot be overstated. High-quality, reliable data is crucial for making accurate estimations and informed decisions. Many case studies indicate that when data is compromised, the resulting assessments can mislead decision-making processes.
Second, collaboration across disciplines enhances the richness of quantitative assessments. Cases that involved multidisciplinary teams often produced better methodologies and more robust findings. For instance, combining forestry expertise with data science has led to the development of advanced models, improving accuracy in predictions.
Additionally, continuous adaptation and methodological advancements are essential. As technology evolves, so too does the capacity for more effective quantitative assessments. Previous case studies show that methods that were once adequate may need reconsideration as new technologies emerge. Keeping abreast of these developments allows for more innovative approaches to managing forest resources.
Quantitative Assessment and Policy Implications
Quantitative assessment in forestry plays a critical role in shaping effective policies for sustainable management of forest resources. By employing rigorous methodologies, a robust analysis of data can emerge, leading to informed decisions. Understanding these processes aids policymakers, forestry professionals, and stakeholders in crafting strategies that balance environmental concerns with economic interests. The central premise is simple β data-driven insights are invaluable for addressing the complex challenges of forestry today.
One significant aspect is the influence of quantitative analysis on sustainable management policies. Accurate measurements of forest health and biomass are essential for determining sustainable yield levels. When policymakers understand the forest's current state through quantitative metrics, they can make rules that safeguard ecosystems while allowing appropriate resource extraction and use. For instance, if assessments show declining biomass in certain regions, immediate actions can be implemented to mitigate further damage. This ability to rely on numerical evidence strengthens the credibility of proposed policies.
Influencing Sustainable Management Policies
Quantitative assessment directly affects sustainable management policies in various ways. It ensures that resource utilization aligns with ecological carrying capacity. Key benefits include:
- Improved Policy Formulation: Data gleaned from quantitative assessments allows authorities to design policies that reflect actual conditions rather than assumptions.
- Targeted Interventions: Policymakers can pinpoint specific areas in need of attention, whether due to over-exploitation, disease, or climatic changes. This precision reduces wastefulness in resource allocation.
- Monitoring Impact: Continuous assessment facilitates the evaluation of policy effectiveness over time, enabling adaptations as necessary to meet changing environmental conditions.
Moreover, if management policies stem from quantitative insights, they can lead to lasting improvements in forest conditions.
Encouragement of Eco-Friendly Practices
The application of quantitative assessment fosters eco-friendly practices in the forestry sector. By enabling a deeper understanding of the interactions within forest ecosystems, forest managers can promote practices that are not only sustainable but also beneficial to biodiversity. This encouragement is evident in the following ways:
- Promoting Best Practices: Quantitative data helps in identifying and sharing successful low-impact logging methods that maintain forest integrity.
- Supporting Certification Programs: Many eco-labeling initiatives rely heavily on detailed assessments to verify that forest products are sustainably sourced. Quantitative evaluations support claims of sustainability, which in turn can enhance market opportunities for products that adhere to stricter standards.
- Enhancing Public Awareness: When data on forest health is made available, it can educate the public about the importance of sustainable practices, ultimately driving consumer demand for eco-friendly products.
"Utilizing quantitative assessments leads to more than just regulatory compliance; it creates a culture of responsibility within the forestry sector." - Forest Policy Expert
Future Directions in Quantitative Assessment
Quantitative assessment in forestry plays a vital role in ensuring sustainable forest management and resource utilization. As environmental concerns grow, so too does the demand for accurate and reliable data. Future directions in this field spotlight emerging trends, innovations, and the integration of advanced technologies. Emphasis on these areas will bolster our understanding of forests and enhance strategies for their conservation and management.
Research Trends and Innovations
Research trends are continually shaping the landscape of quantitative assessment in forestry. One such trend is the increasing application of remote sensing technologies. These advancements enable the collection of vast amounts of data from satellites, drones, and ground-based sensors. This provides critical insights into forest health, biomass estimation, and overall ecosystem functionality.
Another promising area is the development of new statistical methods tailored for ecological data. Traditional models often fall short in addressing the complexity and variability of forest ecosystems. Thus, innovative approaches, such as Bayesian statistics and machine learning algorithms, are gaining traction. These methods allow for more nuanced analyses, improving predictive capabilities and informing management practices.
Finally, interdisciplinary collaboration is on the rise. Forestry professionals are increasingly working with data scientists, ecologists, and technologists to create comprehensive strategies for forest assessment. This diversified approach leads to richer data interpretation and more effective conservation solutions.
The Evolving Role of Data Analytics
Data analytics is transforming the way forestry professionals operate. With vast amounts of data generated from various sources, having robust analytics capabilities is essential. Data analytics provides the tools needed to sift through and make sense of this information. This leads to more informed decision-making processes in forest management.
Furthermore, predictive analytics is becoming essential for anticipating changes in forest conditions. By analyzing historical data, professionals can forecast trends and potential crises, such as pest invasions or climate impacts. Early warnings allow for timely intervention, potentially mitigating losses.
"The integration of data analytics in forestry permits a proactive rather than reactive management approach, greatly enhancing sustainability efforts."
In addition, visualization tools play a significant role. They help stakeholders, including landowners and policymakers, understand complex data more effectively. Graphs, heat maps, and interactive dashboards facilitate communication and foster collaboration among various groups involved in forest management.
The Ends
The conclusions section serves as a critical summary of the entire exploration into quantitative assessment in forestry. It encapsulates the essence of what has been discussed, tying together various methodologies, their applications, and the implications for sustainable forestry practices. Importantly, this section not only summarizes but also emphasizes the significance of the study for practitioners, policy makers, and environmental custodians.
By reflecting on the methodologies employed and the findings derived from quantitative assessments, readers can appreciate how data-driven insights enhance forest management strategies. This section helps in distilling complex information into digestible conclusions, making it easier for professionals to apply what they have learned. In addition, it encourages future investigations into areas that need further exploration, fostering innovation and improved practices in forestry.
Summary of Key Points
- Quantitative assessments are vital for understanding and managing forest resources effectively.
- Statistical models and data analysis tools provide reliable insights into forest health and biomass estimation.
- Advanced technologies, including remote sensing and GIS, improve data accuracy and accessibility.
- Challenges such as data quality must be monitored to maintain the integrity of assessments.
- The results of these assessments influence policy decisions that promote sustainable forestry practices.
Final Thoughts on Quantitative Assessment in Forestry
The future of forestry depends heavily on how well practitioners can utilize quantitative assessment methods. The integration of statistical and technological advancements can significantly enhance the precision of forest evaluations. As environmental challenges become more pressing, the ability to make data-informed decisions is becoming crucial. Thus, forestry professionals are encouraged to adopt these methodologies in their practices. This not only aids in the sustainability of forests but also aligns with broader ecological goals. Continuous learning and adapting to new research trends is essential. By investing in quantitative assessments, we can ensure that forest ecosystems thrive for generations.
"In forestry, effective management is rooted in accurate assessmentβonly then can sustainable practices truly take root."
Investing time and resources into refining these quantitative techniques remains imperative for ensuring our forests are preserved and enhanced, balancing ecological health with human needs.