Understanding the ECMWF Model for Weather Forecasting
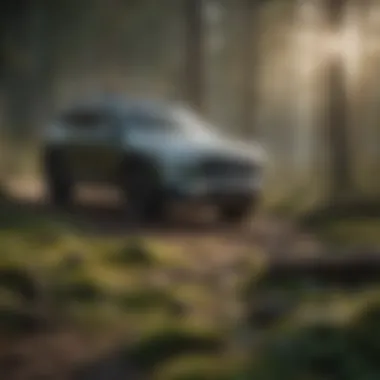
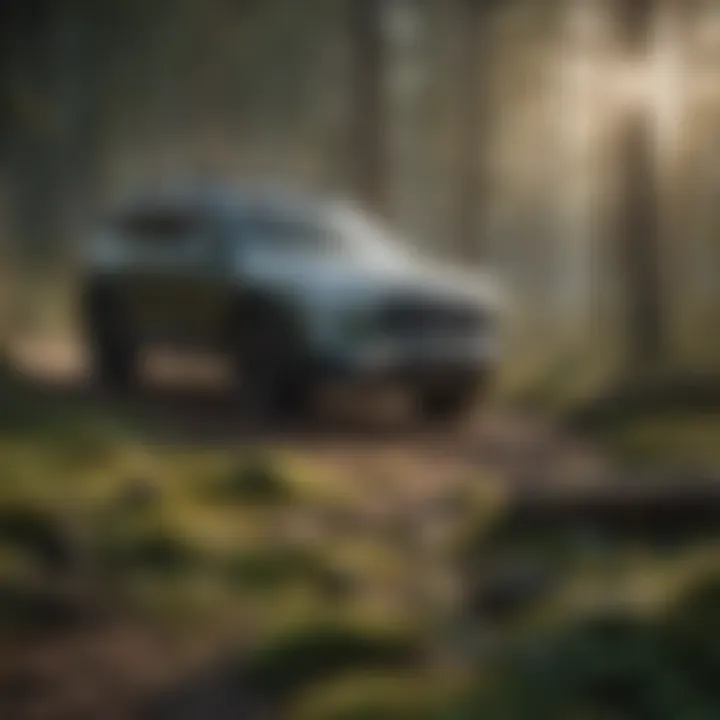
Intro
In today’s complex environment, the integration of weather forecasting and forestry management has emerged as a critical focal point. Specifically, the ECMWF model, known for its reliable predictions, serves as a bridge between meteorological data and sustainable forestry practices. Understanding this model is essential not just for achieving accurate weather forecasts, but also for empowering forest management strategies that are resilient and environmentally responsible. This article aims to dissect the ECMWF model, highlighting its contributions to forestry while also discussing its broader implications on climate research and environmental stewardship.
Overview of Forestry Practices
Importance of Foresting
Forests play a vital role in our ecosystems. They act as the lungs of the Earth, absorbing carbon dioxide and releasing oxygen. In addition to this, forests provide habitat for wildlife, support biodiversity, and enhance water quality. Understanding the significance of forests is paramount for professionals in the sector. Without healthy forest ecosystems, the global climate system is jeopardized.
Types of Forestry Practices
Different forestry practices cater to various ecological and economic needs. Here are few prominent types:
- Commercial Forestry: Focuses on profit-driven timber production, utilizing methods that maximize yield.
- Sustainable Forestry: Aims for responsible management to ensure forests can meet current and future generations' needs.
- Agroforestry: Combines agriculture and forestry, optimizing land use while promoting ecological balance.
- Conservation Forestry: Strives to protect and restore ecosystems by practicing minimal intervention.
Best Practices for Sustainable Woodland Stewardship
Principles of Sustainable Forestry
Embracing sustainable principles is a non-negotiable aspect of forestry management. It includes:
- Ecosystem Integrity: Protecting natural habitats and biodiversity is crucial.
- Economic Viability: Ensuring that forestry practices are economically sound.
- Social Equity: Acknowledging and respecting the rights of local communities.
These principles guide forestry professionals in making informed decisions that benefit both the environment and society.
Techniques for Land Management
Effective land management is pivotal in sustaining forestry. Techniques often involve:
- Selective Logging: Only certain trees are harvested, minimizing environmental impact.
- Reforestation: Planting trees in deforested areas helps restore ecosystems.
- Fire Management: Controlled burns can reduce the risk of uncontrolled wildfires.
Implementing these techniques can ensure the long-term health of forested lands.
Innovative Techniques in Forestry
Latest Technological Advancements
The fusion of modern technology with traditional forestry practices has led to groundbreaking progress. For instance:
- Drones: Used for aerial surveys, mapping, and monitoring forest health.
- Remote Sensing: Enables the collection of data over large areas, improving management efforts.
- Predictive Modelling: Advanced algorithms and ECMWF forecasts assist in anticipating weather patterns that affect forestry dynamics.
Integrating Traditional Knowledge with Modern Science
There is immense value in marrying traditional forestry practices with contemporary science. Local knowledge brings insights that data alone cannot. The combination provides a holistic understanding of land use, ensuring practices are culturally appropriate and environmentally sound.
"Combining local wisdom with scientific inquiry can lead to sustainable solutions that serve not just today, but also tomorrow."
Navigating future challenges in forestry will require adaptive practices rooted in both perspectives.
Overview of the ECMWF Model
The ECMWF model stands out as a cornerstone within meteorology, playing a pivotal role in modern weather forecasting. Understanding it is not merely an academic exercise; it's a necessity for anyone involved in related fields like environmental science, agriculture, or disaster management. At the heart of its significance lies the model's ability to synthesize vast quantities of data and produce reliable predictions, even extending into medium-range forecasting. Essentially, the ECMWF model is a state-of-the-art system that incorporates advanced mathematics and computer science to interpret weather phenomena.
Historical Context
The roots of the ECMWF can be traced back to the early 1970s. Initially established to provide reliable medium-range weather forecasts, it has evolved significantly since its inception. The model's historical development reflects broader trends in technological advancements in meteorology, ranging from rudimentary computer systems to today’s supercomputers capable of processing terabytes of data in seconds. In these past decades, international collaboration has played a crucial role, with European countries pooling resources and expertise. This partnership not only fortified the accuracy of forecasts but also enhanced our understanding of global weather patterns. The model's evolving capabilities underscore its significance in addressing climate change challenges, a concern more prominent than ever in today’s environmental discourse.
Core Objectives
The core objectives of the ECMWF model are multi-faceted yet interlinked. They include:
- High-Quality Forecasting: One of the primary aims is to generate accurate weather predictions for up to 15 days in advance, a feat that is essential for various sectors like agriculture and disaster management.
- Climate Monitoring: Beyond weather forecasting, the ECMWF model also doubles as a tool for climate research, providing critical insights into long-term trends and anomalies.
- Public Safety: By delivering timely and reliable forecasts, the model assists in hazard mitigation, ensuring timely responses to extreme weather events like hurricanes or floods.
- Innovation in Technology: The commitment to integrating cutting-edge technologies, including artificial intelligence and robust data assimilation techniques, drives continuous enhancement of forecast quality.
Ultimately, understanding these objectives allows professionals in related fields to leverage the strengths of the ECMWF model effectively. Its application not only aids in immediate decision-making but also fosters the implementation of long-term sustainability practices. Weather forecasting is no longer just about predicting rain; it's about proactive planning and strategic management.
"The ECMWF is not just a forecasting tool; it's a lifeline for communities facing the unpredictability of climate impacts."
Grasping these elements offers deeper insights into why the ECMWF model commands authority in meteorological circles. The unfolding narrative of this model encapsulates a journey towards precise, responsible, and innovative weather prediction and climate research.
Mechanics of the Model
When it comes to understanding the ECMWF model, the mechanics involved play a crucial role. This section provides an overview of how the model operates and the various components that contribute to its prowess in meteorology. By grasping these mechanical aspects, Forestry professionals and academics can better appreciate the model’s predictive capabilities and its applications across different sectors.
Mathematical Foundations
At the heart of the ECMWF model lies a complex tapestry of mathematical principles. These principles are indispensable for simulating atmospheric processes. The equations employed tend to be the equation of motion and thermodynamics, which intricately describe how air moves and how energy is exchanged.
Statistical methods also come into play—these help to decipher patterns from the massive datasets collected from observations. From differential equations that govern fluid dynamics to numerical methods for solving these equations, it’s a mix of established theories and innovative approaches. This mathematical framework allows the ECMWF model to churn out predictions that, although not foolproof, often hit the nail on the head.
Key takeaway: The foundational mathematics is the backbone of the ECMWF model, enabling reliable forecasts.
Data Assimilation Techniques
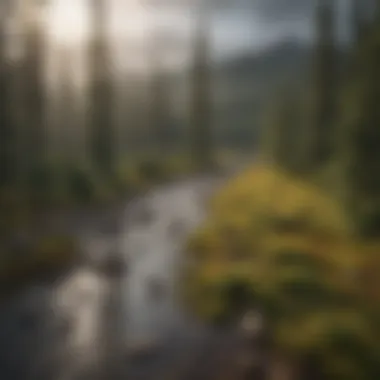
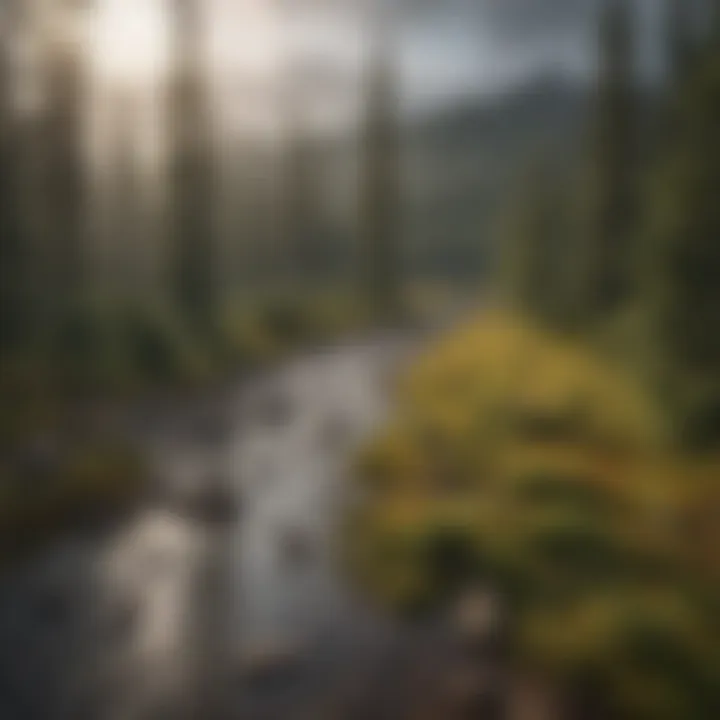
Data assimilation techniques are what make the ECMWF model truly shine. These methods serve as a bridge between the raw data collected from various sources and the actual model used for predictions. In simpler terms, it’s all about blending observed data with the model’s current state to produce a more accurate forecast.
The ECMWF employs a sophisticated system called the "4D-Var" (four-dimensional variational) data assimilation. Through this technique, the model takes into account not just the current observations but how those observations evolve over time. By doing this, it effectively minimizes the differences between predicted and observed states, enhancing the reliability of its forecasts significantly.
Here are some key steps involved in data assimilation:
- Collection of observations from satellites, weather stations, and buoys.
- Use of algorithms to adjust the model’s initial conditions based on the incoming data.
- Continuous recalibration of the model as new information becomes available.
This process underscores the importance of accurate real-time data in achieving reliable weather forecasts.
Numerical Weather Prediction
Numerical weather prediction (NWP) is where theory meets real-world applications. The ECMWF model utilizes numerical methods to simulate the atmosphere's behavior. It breaks down the atmosphere into a grid, where each point on the grid represents a specific volume of air. The model then applies mathematical equations to predict how the properties of each grid point change over time.
The result? A detailed forecast that encompasses various parameters including temperature, humidity, and wind speed. NWP allows meteorologists to provide short, medium, and long-range forecasts that are vital for numerous sectors, including agriculture, disaster management, and environmental research.
Common forms of numerical methods include:
- Finite difference methods: Useful for solving differential equations across the grid.
- Perturbation methods: Apply small changes to initial conditions to see how predictions vary.
In summary, the mechanics of the ECMWF model hinge on a delicate balance of mathematical principles, clever data assimilation techniques, and robust numerical methods, all of which contribute to its influential role in weather forecasting.
The Role of Observations
Observations form the backbone of the ECMWF model. They are the lifeblood that nourishes the system, enabling it to translate raw data into meaningful forecasts. Observations can be thought of as a wide-ranging language of the atmosphere, capturing its various nuances and complexities. In this section, we delve into the significance of observations, emphasizing the integral roles they play in enhancing the model's efficacy and precision.
Satellite Data Utilization
Satellite data represent a revolutionary advancement in meteorology. They offer a vantage point high above the Earth's atmosphere, providing a sweeping view that ground-based observations simply cannot match. Satellites are equipped with a host of instruments that measure various parameters such as temperature, humidity, winds, and cloud cover. This real-time data flow is crucial for modeling weather patterns accurately.
- Global Coverage: Satellites provide extensive coverage across vast regions. Unlike ground stations, which can be sparsely located, satellites orbit the Earth, collecting data from remote areas, such as oceans and polar regions, thus painting a more complete picture of global weather systems.
- Timeliness: The frequency at which satellite data is gathered allows the ECMWF model to respond dynamically to real-time weather changes. This responsiveness improves medium-range forecasting precision by updating the model more frequently with new data inputs.
- Advanced Sensors: Modern satellites carry sophisticated sensors that can penetrate cloud cover, providing crucial information about the state of the atmosphere even when visibility is low. For instance, the European Space Agency's Copernicus program uses Sentinel satellites to monitor environmental changes, delivering data that significantly enhance forecasting accuracy.
The use of satellite data in weather modeling cannot be overstated; it enhances not only the precision of forecasts but also our understanding of climate dynamics that may influence forestry and agricultural practices.
Because satellite data is crucial, the ECMWF continually refines its data assimilation techniques to integrate this information effectively. This ensures that even the subtle clues from the atmospheric behaviors are accounted for, enhancing the model’s predictive power.
In-situ Measurements
While satellite data offers a grand overview, in-situ measurements provide the ground-level detail that is equally vital. These observations are collected directly from weather stations, buoys, and other instruments located on the surface. In-situ measurements are essential for validating satellite data and provide local insights that can sometimes be lost from high-altitude observations.
- Localized Data Collection: Ground-based sensors are positioned strategically to offer concentrated insight into local climatic conditions. For instance, a weather station in a forested area can provide data about humidity and temperature variations that significantly impact growth and health of trees in that specific region.
- Integration with Other Data: By coupling in-situ measurements with satellite data, the model's accuracy receives a substantial boost. The combination creates a more nuanced picture of weather phenomena. With this synergy, complications such as microclimates—areas with weather patterns that differ from the surrounding regions—are effectively managed in modeling processes.
- Quality Control: Ground measurements also serve as a crucial checkpoint. They assist in confirming the reliability of satellite data, ensuring that the model bases its predictions on accurate parameters. Discrepancies between in-situ and satellite data can highlight errors or areas needing improvement in the observational network.
In summary, observations—both satellite and in-situ—are fundamental to the ECMWF model. They provide the necessary raw data that feeds into numerical predictions, allowing this sophisticated tool to function effectively. By integrating a diverse range of observational data, the model can deliver forecasts that serve various sectors, including forestry management, ensuring a more sustainable relationship with the environment.
Forecasting Capabilities
Forecasting capabilities are pivotal within the ECMWF model framework, molding its effectiveness in delivering weather insights that influence decision-making across sectors. The precision of medium-range forecasts, the foresight in seasonal predictions, and the wide reach of long-term climate projections form the triad of forecasting elements that underscore the model's value. Each segment serves not only to enhance operational methodologies but also to address growing concerns around climate variability and change, making understanding this section crucial for forestry professionals and academics alike.
Medium Range Forecasting
Medium range forecasting, generally spanning around 3 to 15 days ahead, stands as a testimony to the ECMWF model's adeptness in harnessing data for timely prediction. This time frame positions it as a bridge between short-term forecasts, which often lack in depth, and long-term projections that hinge on broader climatological patterns. The implications for sectors like agriculture and forestry are significant; for instance, knowing potential rainfall or temperature fluctuations can help farmers optimize crop management and mitigate losses from unexpected weather events.
Notably, the ECMWF model leverages sophisticated data assimilation techniques, incorporating observations from satellites and ground-based measurements to refine its predictions. The accuracy of these forecasts has improved remarkably over recent years, as the model continuously integrates feedback from previous forecasts, allowing it to learn and adapt. Therefore, practitioners in agriculture and related fields find immense value in medium-range forecasts to make informed operational decisions.
Seasonal Predictions
Seasonal predictions extend the foresight of the ECMWF model beyond the immediate horizon, typically ranging from one to six months. This capability is particularly beneficial for industries that operate on longer time scales, such as forestry or agriculture, where understanding seasonal weather patterns can dictate planning and resource allocation.
What sets the ECMWF model apart is its ability to combine various data sources, including ocean temperature patterns, atmospheric circulation models, and historical data. By recognizing patterns that recur with seasonal cycles, the model helps stakeholders anticipate weather phenomena like El Niño or La Niña, which can drastically influence precipitation and temperature on a regional scale.
"The ability to prepare for and mitigate climate effects through seasonal predictions cannot be overstated; it aligns with sustainable practices that benefit both the environment and the economy."
Thus, seasonal predictions offer an essential perspective for preparing for weather patterns that could affect crop yields or forest health, allowing for strategic adaptations in management practices.
Long-term Climate Projections
Long-term climate projections play a crucial role in shaping climate adaptation strategies and environmental policy. These projections often look ahead multiple decades, providing insights into evolving climate scenarios driven by factors like greenhouse gas emissions, land-use changes, and natural climate variability. For sectors like forestry, understanding these trends is indispensable for developing robust conservation strategies and sustainable management practices.
The ECMWF model utilizes complex simulations to forecast how climate conditions will alter over time. This is not simply about guessing the weather next weekend or even next summer; it involves projecting broader shifts that could transform ecosystems, alter species distributions, and affect carbon storage capabilities.
Stakeholders can harness these projections to evaluate potential impacts on biodiversity, forest health, and regenerative capabilities. Moreover, by using this information, policymakers can initiate long-term strategies to counter adverse impacts, promoting resilience against climate variability.
Applications in Various Sectors
The ECMWF model is more than just a sophisticated meteorological tool; it significantly impacts various sectors by offering valuable forecasts and insights. Understanding these applications is key to appreciating how weather predictions influence different industries and societal functions. This section explores how the ECMWF model finds relevance in agriculture, disaster management, and environmental research, emphasizing both its innovative contributions and the challenges that come with it.
Agriculture Impact Assessment
Crop Yield Predictions
Crop yield predictions are vital for sustainable agricultural practices, and the ECMWF model offers a framework for making informed decisions. By leveraging accurate weather forecasts, farmers can better predict the ideal planting times, monitor growth conditions, and ultimately enhance productivity. The ability of the ECMWF model to integrate data over different time frames allows for dynamic monitoring of various crop types, adapting to conditions as they unfold.
Another key characteristic of these predictions is their adaptability. They can help tailor agricultural strategies based on real-time weather data, which is incredibly beneficial in today's fast-paced, climate-affected agricultural environment. A unique feature of these predictions is the incorporation of various scenarios, allowing stakeholders to prepare for diverse outcomes. However, reliance on predictions can also lead to overconfidence, especially in unpredictable weather conditions, so taking the forecasts with a grain of salt is essential.
Weather-related Risks
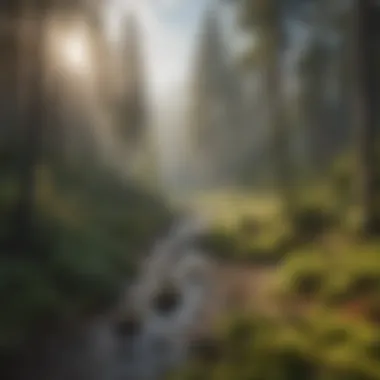
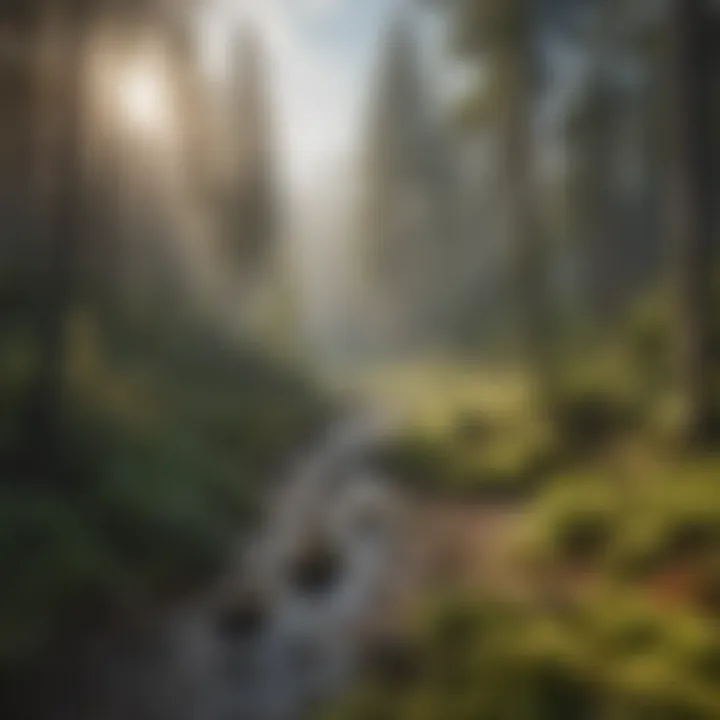
Weather-related risks pose significant threats to agricultural operations, and the ECMWF model's ability to accurately forecast inclement weather plays a critical role in risk mitigation. These forecasts help farmers anticipate adverse weather events, such as hailstorms or unexpected frosts, which could ruin crops overnight. The essence of these predictions lies in assessing risks early enough to implement preventive measures.
The key characteristic of addressing weather-related risks through the ECMWF model is the timely issuance of alerts, which are invaluable for planning and strategy. This value is seen in how farmers can deploy resources effectively based on forecasts. While the advantages are clear, like improved resilience against climate variability, there can be a downside as well—overreliance on forecasts may lead to complacency with standard operational practices.
Disaster Management
Flood and Drought Monitoring
The role of the ECMWF model in monitoring floods and droughts has become indispensable. By providing critical data, it enables governments and organizations to assess water availability and track moisture levels in the soil, allowing for proactive measures before disasters strike. Monitoring capabilities translate directly into informed decision-making, which can save lives and livelihoods.
One of the strong points of flood and drought monitoring through the ECMWF model is its capacity for long-range forecasting combined with high-resolution data. This combination enhances the accuracy of predictions, making it easier to implement emergency preparedness plans. A unique challenge, however, is the inherent uncertainty in long-range forecasts, which can affect the reliability of the information presented in real-world applications.
Emergency Response Planning
When it comes to emergency response planning, the ECMWF model stands as a cornerstone for devising effective plans. The model assists organizations in preparing for possible scenarios resulting from severe weather events. Whether it’s coordinating evacuations or allocating resources in advance, the model’s simulations have proved to be a strategic asset.
Emergency response planning benefited significantly from the ECMWF model's tools, which help visualize various potential impacts of weather events. The unique feature here is the model's ability to run simulations based on historical data combined with projected weather patterns, empowering authorities to make well-informed decisions. Yet, there exists a crucial consideration: sometimes, forecasts can lead to false alarms, causing unnecessary panic or resource wastage.
Environmental Research
Environmental research greatly benefits from ECMWF outputs, enabling scientists to study climate change impacts, biodiversity, and more. The model supplies crucial data that aids in modeling ecological changes and informs policy decisions. By analyzing weather patterns, researchers can better understand phenomena, offering deeper insights into how climate influences ecosystems.
In sum, the ECMWF model is an invaluable resource across sectors, offering a comprehensive tool for decision-making and strategic planning. Its applications may vary, but they all hinge on the core principles of information gathering and predictive analytics, facilitating better responsiveness in crucial areas.
Technological Innovations
Technological innovations are at the heart of advancing predictive capabilities in the ECMWF model. As the demands for accurate weather forecasting escalate, integrating cutting-edge technologies is no longer optional. Instead, it has become a cornerstone of reliable meteorological practices. The innovations within this domain serve various crucial functions, from improving data accuracy to enhancing computational efficiency.
Artificial Intelligence Integration
Artificial Intelligence has found its way into meteorological models, transforming how forecast data is processed and interpreted. In the case of the ECMWF model, AI techniques assist in identifying patterns and making predictions that were previously beyond reach. For instance, machine learning algorithms analyze historical weather data, enabling the model to adapt and refine its forecasting processes.
- Benefits of AI in Weather Forecasting
- Pattern Recognition: AI can sift through vast datasets to uncover patterns that may not be visible to human analysts.
- Predictive Analytics: Leveraging complex statistical models, AI enhances forecasting accuracy by forecasting potential weather scenarios.
- Real-time Processing: The ability to analyze information in real-time allows for quicker updates to forecasts, which is vital for time-sensitive situations like severe weather alerts.
However, there is a caveat. As much as AI improves efficiency, it also introduces challenges such as data bias and the need for transparency in algorithms. It is essential to maintain an ethical standard in how these technologies are applied.
High-Performance Computing
High-performance computing plays an indispensable role in the ECMWF model, enabling it to handle massive datasets and perform intricate calculations quickly. The sheer volume of meteorological data generated each day is staggering, from satellite observations to ground-level instruments. Without high-performance computing, processing this data could take an impractical amount of time.
- Key Aspects of High-Performance Computing:
- Speed: Advanced computing resources enable faster data processing and quicker turnaround on forecasts.
- Complexity: Solving highly complex mathematical models that simulate atmospheric processes needs significant computational power, achievable only through supercomputers.
- Scalability: With continuous advancements in technology, high-performance computing can easily scale as data collection methods evolve.
"High-performance computing has revolutionized how we approach weather forecasting, providing us with the ability to predict with more confidence and speed."
The benefits brought on by these technological advancements ultimately enhance the ECMWF model's utility across different sectors, solidifying its position as a leading tool in weather forecasting. The blend of AI and high-performance computing is not just an upgrade but a transformative leap that allows meteorologists to tackle the complexities of weather with greater precision.
Challenges and Limitations
In the context of the ECMWF model, navigating through its challenges and limitations is not just a mere academic exercise; it's essential for understanding its overall efficacy in weather forecasting. The model’s capabilities are undoubtedly impressive, but like any predictive framework, it isn't without its flaws. Recognizing these obstacles helps refine the model’s functionality and guides users in interpreting forecast results more effectively.
Model Uncertainty
Model uncertainty is often viewed as a double-edged sword in the realm of meteorological forecasting. This uncertainty arises from a variety of sources, including mathematical approximations inherent in the model and the assumptions made during data assimilation processes. For example, the complexity of atmospheric processes and incomplete knowledge of initial conditions can lead to discrepancies in the predicted outcomes.
Understanding how uncertainty manifests can empower meteorologists to make more informed assessments of forecast reliability. Users who rely on ECMWF predictions must acknowledge that while the model is robust, it can’t guarantee absolute accuracy. For instance, during particularly volatile weather patterns like hurricanes, model uncertainty may lead to variations in storm track predictions, creating challenges for emergency planning.
When interpreting forecasts, the following points are essential to keep in mind:
- Probabilistic Forecasting: Many ECMWF outputs include probabilities of various weather scenarios, helping users grasp the range of possible outcomes.
- Confidence Intervals: Understanding the confidence intervals associated with predictions can provide insight into the model's reliability.
- Case Studies: Reviewing past forecast errors helps identify patterns and improves future model results.
"Forecasting the future of weather is like trying to catch a greased pig; it’s slippery and unpredictable."
Sensitivity to Initial Conditions
Another significant challenge facing the ECMWF model, and indeed all numerical weather prediction models, is its sensitivity to initial conditions. This concept, famously illustrated by the butterfly effect, posits that even minute variations in the starting point of a simulation can lead to vastly different outcomes down the line. Essentially, if the initial atmospheric conditions are slightly off, the forecast can spiral into a completely incorrect trajectory.
This sensitivity necessitates a heightened focus on the quality and precision of initial data inputs. For instance, if an incorrectly calibrated sensor reports the temperature by just a degree, this could lead to a significant deviation in the following forecast. It underscores why continual investment in high-quality observational data and advanced assimilation techniques is vital for model fidelity.
Adding to the complexity, meteorological phenomena displaying chaotic behavior can magnify these discrepancies. For example, short-term forecasts can perform exceptionally well, but as the period extends, the reduction in accuracy becomes evident unless the initial conditions were perfectly aligned.
Key aspects to consider about sensitivity include:
- Data Quality: Ensuring that the initial conditions are as accurate as possible is paramount.
- Ensemble Forecasting: Running multiple simulations with varied initial conditions can help gauge the uncertainty bounds of forecasts.
- Regular Updates: Continuous updates to the input data are crucial to improve predictability, especially in dynamically changing atmospheric scenarios.
In summary, while the ECMWF model serves as a powerful tool for weather forecasting, grappling with these challenges—model uncertainty and sensitivity to initial conditions—is essential. Addressing these areas not only enhances the model’s reliability but also strengthens the user’s ability to apply forecasts effectively.
Regulatory and Ethical Considerations
In a world increasingly reliant on data and analytics, the regulatory and ethical landscape surrounding the use of meteorological models like the ECMWF becomes paramount. This section explores how these considerations not only shape the operational framework of the model but also affect its widespread applicability across various sectors. The responsible use of data and adherence to ethical standards can significantly enhance the credibility and effectiveness of the forecasts produced by the ECMWF model.
Data Privacy Concerns
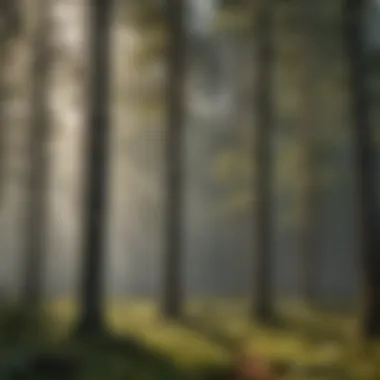
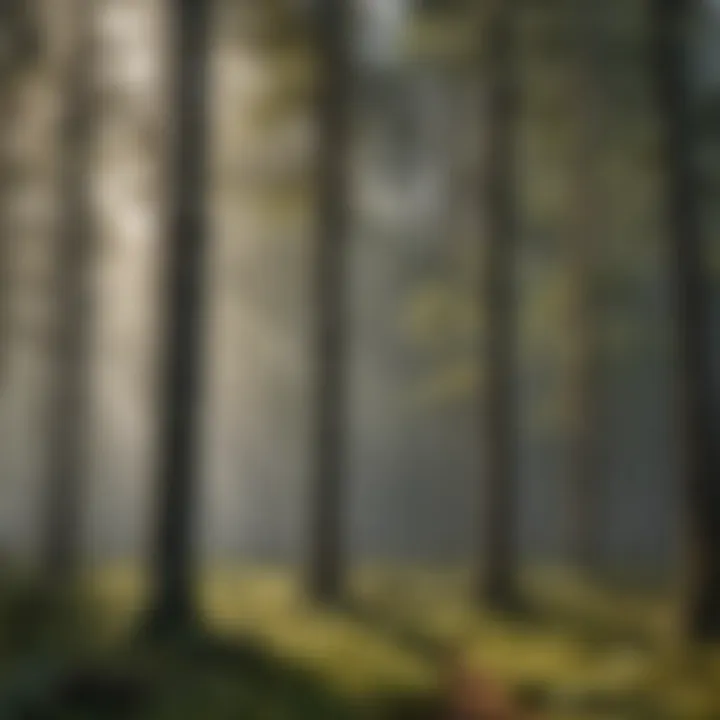
Data privacy is a top-tier concern in today's digital environment, and it holds particular weight when it comes to meteorological forecasting, where vast amounts of data are collected and analyzed. The ECMWF model processes both satellite-derived data and in-situ observations, often involving personal or location-specific information.
The key issues around data privacy include:
- Consent and Anonymity: Individuals often don't realize when their data is being used in predictive models. Proper mechanisms should be in place to ensure data collection is conducted with informed consent, and any personal identifiers must be anonymized to protect individual privacy.
- Data Management: With so much data flowing in from various sources, it is crucial for organizations to employ robust data management practices, ensuring that sensitive information is securely stored and accessed only by authorized personnel.
- Regulatory Compliance: Laws such as the General Data Protection Regulation (GDPR) in the European Union set stringent requirements for handling personal data. The ECMWF must ensure compliance with such regulations to avoid legal repercussions and foster public trust.
To mitigate these issues, organizations can adopt practices such as regular audits and establishing transparent policies regarding data utilization, creating a culture of accountability and respect for privacy.
Usage in Public Policy
The ECMWF model is not just a tool for predicting the weather; it has significant implications for public policy, particularly in areas like environmental protection, disaster management, and infrastructure planning. However, its application in policymaking brings various ethical considerations to light.
Some critical points include:
- Guiding Governmental Decisions: Accurate weather forecasts can guide crucial decisions in various sectors. Policymakers depend on the ECMWF model for information that can influence everything from emergency preparedness to resource allocation in agriculture.
- Public Accessibility: As forecasts often dictate life and safety measures, it is essential for the information derived from the ECMWF model to be accessible to the public. Transparency in how models are developed, how forecasts are made, and how the data is utilized helps build trust in governmental recommendations.
- Equitable Use of Information: Ensuring that all communities have equal access to forecasting information is vital. Disparities in data access may lead to inequities, especially for vulnerable populations who may be more adversely affected by natural disasters and climate phenomena.
"Effective public policy is not just about robust data; it's also about ensuring that the insights are disseminated and used ethically to benefit all citizens."
Addressing these ethical dimensions in the application of the ECMWF model can lead to more inclusive and effective policymaking, ultimately supporting sustainable development goals.
Future of the ECMWF Model
The future of the ECMWF model stands as a pivotal point of anticipation in the field of meteorology. As the climate continues to change and the demands for precise weather forecasting grow, the evolution of this model will likely integrate new methodologies and technologies. In understanding its trajectory, we can better appreciate its role not only in routine weather prediction but also in broader climate assessments that affect sustainability across various sectors, notably in forestry.
advancements in modeling techniques, computational capabilities, and data integration will directly influence the ECMWF model’s performance. Continuous improvement in these areas is essential for addressing the escalating challenges posed by climate variabilities. The model's ability to assimilate more diverse data sources could enhance its reliability. This brings us to explore the emerging trends and prospective enhancements that will shape the ECMWF model.
Emerging Trends in Meteorology
The landscape of meteorology is undergoing transformations that are likely to redefine traditional practices and theories. For instance, one prominent trend is the incorporation of machine learning algorithms into forecasting practices. These algorithms harness voluminous datasets, enabling more nuanced predictions that extend beyond simple statistical methods. As models learn from past weather patterns, their predictive abilities sharpen with time, creating an almost intuitive foresight into climactic shifts.
You know how sometimes you just feel the weather's changing? That’s a bit how these advancements work. They improve the model’s understanding of patterns, relying less on human interpretation and more on raw data insights.
Moreover, the increasing availability of high-resolution remote sensing data prompts a shift toward micro-scale forecasting. This approach allows for precision in predictions that can significantly impact local communities, particularly in agricultural and forestry sectors.
In addition, collaboration across borders and disciplines is becoming more pronounced. The need for shared data and methodologies in meteorology propels partnerships. Such collaborations foster improvements in ECMWF functionalities, paving the way for innovations that would not have been possible in an isolated framework.
Prospective Enhancements
Looking ahead, we expect the ECMWF model to experience substantial upgrades that will bolster its forecasting accuracy and scope. Key prospective enhancements include:
- Cloud-Computing Power: Leveraging the power of cloud computing ensures not only faster processing times but also the capability to handle vast datasets effectively. This enables the integration of real-time data streams, which can adjust forecasts dynamically.
- Integration of Non-Traditional Data Sources: Utilizing social media and crowd-sourced weather reports could serve to enrich the model’s data pool. This innovative practice allows for anecdotal evidence of weather phenomena that formal reporting mechanisms may miss.
- Long-term Monitoring Capacities: As climate changes, having the ability to project beyond typical forecasting ranges becomes critical. Enhanced models will likely incorporate climate scenarios that can inform forestry practices, helping managers adapt over time.
- Improved Visualization Tools: With advancements in graphic technologies, we expect clearer and more intuitive representations of model outputs. This would help forestry professionals understand potential impacts quickly and apply them to their management strategies.
"The future of the ECMWF model is not just about predicting feels like weather, but understanding the implications of these forecasts on our planet’s health and responsible resource management."
In essence, the amalgamation of these emerging trends and potential enhancements is set to redefine the ECMWF model. As it evolves, it promises to deliver sharper, more actionable insights critical for maintaining equilibrium in forestry practices amid ongoing climatic challenges.
Implications for Sustainable Forestry
The relationship between meteorological models and sustainable forestry practices is both intricate and significant. As the world grapples with the impacts of climate change, understanding the implications of the ECMWF Model on forestry is crucial. The model provides insights into anticipated weather patterns, which can inform the decisions made by forestry professionals across various domains.
Benefits of Integrating Meteorological Insights in Forestry
Working with the ECMWF Model offers several advantages for sustainable forestry:
- Mitigation of Risks: Predicting adverse weather conditions such as droughts or heavy rains aids forestry managers in mitigating risks associated with forest health and productivity. These forecasts can drive proactive measures like adjusting harvest schedules or implementing protective measures.
- Optimization of Growth Conditions: Foresters can utilize sophisticated forecasts to identify optimal planting times and conditions. This is especially important for species that are sensitive to frost or require specific moisture levels.
- Resource Management: Accurate weather predictions enable more effective resource management. For example, knowing when rainfall is expected can inform irrigation strategies in tree nurseries, ensuring that seedlings receive the right amount of water without overusing resources.
Considerations for Effective Implementation
For the effective integration of ECMWF Model data into forestry management, several considerations must be taken into account:
- Training and Capacity Building: Forestry professionals need training on how to interpret and utilize meteorological data for their specific practices. This encompasses understanding the model's limitations and variability in forecasts.
- Data Accessibility: Ensuring that reliable access to ECMWF forecasts is available for forest management practitioners is key. Recommendations and user-friendly interfaces can facilitate easier adoption by non-experts.
- Collaboration: Sustainable forestry efforts can be heightened through collaboration with meteorologists and climate scientists. Their expertise can enrich the understanding of regional climate dynamics and how they interact with forest ecosystems.
"Effective forest management requires an understanding of how weather patterns affect forest dynamics. The ECMWF Model provides a vital tool in this understanding."
In summary, the implications for sustainable forestry rooted in ECMWF data are vast and multilayered. By integrating forecasting models into their practices, forestry professionals stand to enhance the resilience of forest ecosystems, optimize their practices, and contribute to more sustainable resource management.
Impact on Forest Management Practices
In the realm of forestry, the ECMWF Model can profoundly influence management practices. With a keen understanding of weather trends and conditions, forestry managers can make informed decisions that lead to more sustainable outcomes. This section explores how model data translates into actionable practices.
Adaptive Management Strategies
Utilizing forecasts allows for adaptive management strategies that respond more dynamically to changing climatic conditions. Some key impacts include:
- Resilient Species Selection: By analyzing long-term weather patterns, managers can choose tree species and varieties that are likely to thrive under future climate scenarios. This ensures better survival rates and productivity.
- Timely Interventions: Weather forecasts provide critical windows for interventions, such as controlled burns or pest management. Knowing in advance when conditions may be suitable for such actions helps to optimize their effectiveness.
- Monitoring and Assessment: Incorporating model data into regular monitoring allows for earlier detection of changes in forest health. This proactive approach facilitates quicker responses to emerging issues that threaten forest vitality.
Role in Climate Adaptation Strategies
The ECMWF Model also plays a pivotal role in shaping climate adaptation strategies within the forestry sector. As shifts in weather patterns become more pronounced, adapting to these changes is essential for sustainability.
Framework for Adaptation
- Scenario Planning: The ability to project various climate scenarios helps forest managers plan for a range of future conditions. This means they can develop comprehensive strategies addressing potential impacts from shifts in temperature, precipitation, and extreme weather events.
- Project Development: With accurate forecasting, forestry professionals can design projects that integrate climate resilience into their frameworks. This could mean selecting planting sites that are likely to remain viable in the face of future climatic trends.
- Community Engagement: Engaging local communities in discussions about predicted climate changes fosters a collaborative approach to adaptation. It ensures that adaptive strategies are culturally and socially relevant, leading to lasting impacts.
Epilogue and Final Thoughts
As we draw the curtains on this exploration of the ECMWF model, it becomes clear just how crucial this tool is in today’s dynamic environment, especially for forestry professionals and academics. The ECMWF model stands as a pillar of understanding and predicting atmospheric phenomena. Its strengths lie in the sophisticated techniques used for data assimilation and forecasting, which provide invaluable insights across various sectors.
In summarizing the key insights presented throughout this article, it’s evident that the ECMWF model’s historical context and core objectives lay the groundwork for its ongoing evolution. The mechanics detailed, particularly the mathematical foundations, have set the stage for enhanced predictive capabilities. Through advancements in technology, such as artificial intelligence and high-performance computing, the model continues to adapt, tackling new challenges with each passing day.
Moreover, the implications of this model stretch far beyond mere predictions. The discussions around sustainable forestry management showcase its relevance in addressing climate adaptation strategies that businesses and governments grapple with daily. The integration of predictive weather capabilities enables forestry professionals to make informed decisions, ensuring that they respond proactively to environmental changes rather than reactively.
"The forecasts generated by the ECMWF model are not just data—they are lifelines for effective decision-making in forestry and beyond."
Looking ahead, we must consider the future trajectory of the ECMWF model amidst emerging trends and challenges. The prospective enhancements in its capabilities could surely bring about transformative changes, necessitating ongoing research and adaptation in how we use and interpret this model. It invites us to remain vigilant and responsive to the evolving landscape of meteorology and its implications for our world.
Ultimately, as we reflect on the intricate relationship between weather forecasting and the realms of forestry, it’s clear that the ECMWF model is not just a tool but a partner in navigating the complexities of climate and environmental stewardship.